In my previous post (git repo) I ask an LLM to compare the relevance of two products from the WANDS furniture e-commerce search dataset. I then compare the agreement with the preference of human search raters, hoping if I got close to agreement, I might be onto creating a good pairwise LLM search relevance evaluator without so many humans.
I note that when letting the LLM chicken-out and say “I dont know”, precision improves. But naturally reduces the recall - the percentage of labeled product pairs - quite a bit.
So, as an example, we might start with the following, simple, forced decision, asking an LLM to tell us which chair is more relevant to the leather chairs
query:
System: You are a helpful assistant evaluating search relevance of furniture products.
Prompt:
Which of these products is more relevant to the furniture e-commerce search query:
Query: leather chairs
Product LHS: fashion casual work beauty salon task chair
Product RHS: angelyn cotton upholstered parsons chair in gray/white
Respond with just 'LHS' or 'RHS'
Response: LHS
If humans also say “LHS” - the salon task chair - is more relevant, then this is a win!
Of course, in the WANDS case, as with many datasets we don’t have direct pairwise labels. Instead we have absolute labels. We’re really just comparing which human label is higher. That is, on a 0-2 scale, LHS might be labeled by humans a 2 for completely relevant, RHS a 0 for absolutely horrid result for leather chair
, etc. So we say humans see LHS as more relevant.
The above prompt, over 1000 pairs, gives:
poetry run python -m local_llm_judge.main --verbose --eval-fn name
...
Precision: 75.08% | Recall: 100% (N=1000)
But we can also allow the LLM to NOT label a pair due to lack of information:
Neither product is more relevant to the query, unless given compelling evidence.
Which of these product names (if either) is more relevant to the furniture e-commerce search query:
Query: leather chairs
Product LHS name: fashion casual work beauty salon task chair
(remaining product attributes omited)
Or
Product RHS name: angelyn cotton upholstered parsons chair in gray/white
(remaining product attributes omited)
Or
Neither / Need more product attributes
Only respond 'LHS' or 'RHS' if you are confident in your decision
Respond with just 'LHS - I am confident', 'RHS - I am confident', or 'Neither - not confident'
with no other text. Respond 'Neither' if not enough evidence.
Response:
Neither - not confident
Doing this 1000 times gives:
poetry run python -m local_llm_judge.main --verbose --eval-fn name_allow_neither
...
Precision: 85.38% | Recall: 17.10% (N=1000)
Out of the 17.10% labeled, 85.38% agreed with humans.
Adding an important sanity check - checking both ways
Turns out, it’s important to check twice. Put the LHS product on the RHS, and double check that you the same result. In other words ask first: is ‘salon chair’ more relevant than ‘parsons chair’ for query leather chair
? Then reset, swap, and ask that is parsons chair
more relevant than salon chair
? We can then account for any biases an LLM might have to attending to the first or second product listed.
We wrap the prompt (eval_fn
below) in a python function that double checks:
def check_both_ways(query, product_lhs, product_rhs, eval_fn): # eval_fn is a function wrapping the prompt
"""Get pairwise preference from LLM, but double check by swapping LHS and RHS to confirm consistent result."""
decision1 = eval_fn(query, product_lhs, product_rhs) # This just calls the LLM with the prompt LHS to RHS
decision2 = eval_fn(query, product_rhs, product_lhs) # Now check RHS first...
if decision1 == 'LHS' and decision2 == 'RHS':
return 'LHS'
elif decision1 == 'RHS' and decision2 == 'LHS':
return 'RHS'
return 'Neither'
Adding a command line switch check twice:
$ poetry run python -m local_llm_judge.main --verbose --eval-fn name --check-both-ways
This dramatically improves performance. When the above is run, precision goes up appreciability with a high degree of product coverage:
Precision: 87.99% | Recall: 65.80% (N=1000)
Even more interesting, when combining with allowing ‘I dont know’, we get the highest precision. Though with a significant reduction in recall:
$ poetry run python -m local_llm_judge.main --verbose --eval-fn name_allow_neither --check-both-ways
...
Precision: 90.76% | Recall: 11.90% (N=1000)
So to summarize where my efforts stand, just using product name, we can build this confusion matrix. Showing Precision / Recall for each approach:
Dont check | --check-both-ways |
|
---|---|---|
Force | 75.08% / 100% | 87.99% / 65 % |
Allow Neither | 85.38% / 17.10% | 90.76% / 11.90% |
So depending on your use case, you should pick the appropriate solution. Want a high degree of coverage and can tolerate a lot of mistakes. Use the force / dont double check. Want to tolerate very few mistakes and only flag results with real issues? Double check and allow the LLM to say it doesn’t know (ie ‘allow neither’).
I tried creating evaluators looking only at a few other fields. Here’s showing the LLM only product “class” (as in classification). Example product classes:
Product LHS class: Beds
Product RHS class: Kids Beds
Product LHS class: Coffee & Cocktail Tables
Product RHS class: Outdoor Fireplaces
Run with these 4 variants:
poetry run python -m local_llm_judge.main --verbose --eval-fn classs
poetry run python -m local_llm_judge.main --verbose --eval-fn class_allow_neither
poetry run python -m local_llm_judge.main --verbose --eval-fn classs --check-both-ways
poetry run python -m local_llm_judge.main --verbose --eval-fn class_allow_neither --check-both-ways
Dont check | --check-both-ways |
|
---|---|---|
Force | 70.5% / 100% | 87.76% / 58.0% |
Allow Neither | 87.01% / 17.70% | 84.47% / 10.3% |
And repeating for the product’s full categorization hierarchy (like Outdoor furniture > Seating > Adirondak Chairs...
)
Dont check | --check-both-ways |
|
---|---|---|
Force | 74.6% / 100% | 86.1% / 69.70% |
Allow Neither | 85.71% / 18.20% | 89.91% / 10.8% |
Finally, noisiest of them all, product description:
Dont check | --check-both-ways |
|
---|---|---|
Force | 70.31% / 98.70% | 76.58% / 72.60% |
Allow Neither | 79.21% / 10.10% | 83.02% / 5.3% |
(Note with product description even ‘forcing’ a decision the LLM still sometimes said it couldn’t tell, completely unprompted!)
Sign up for my class!
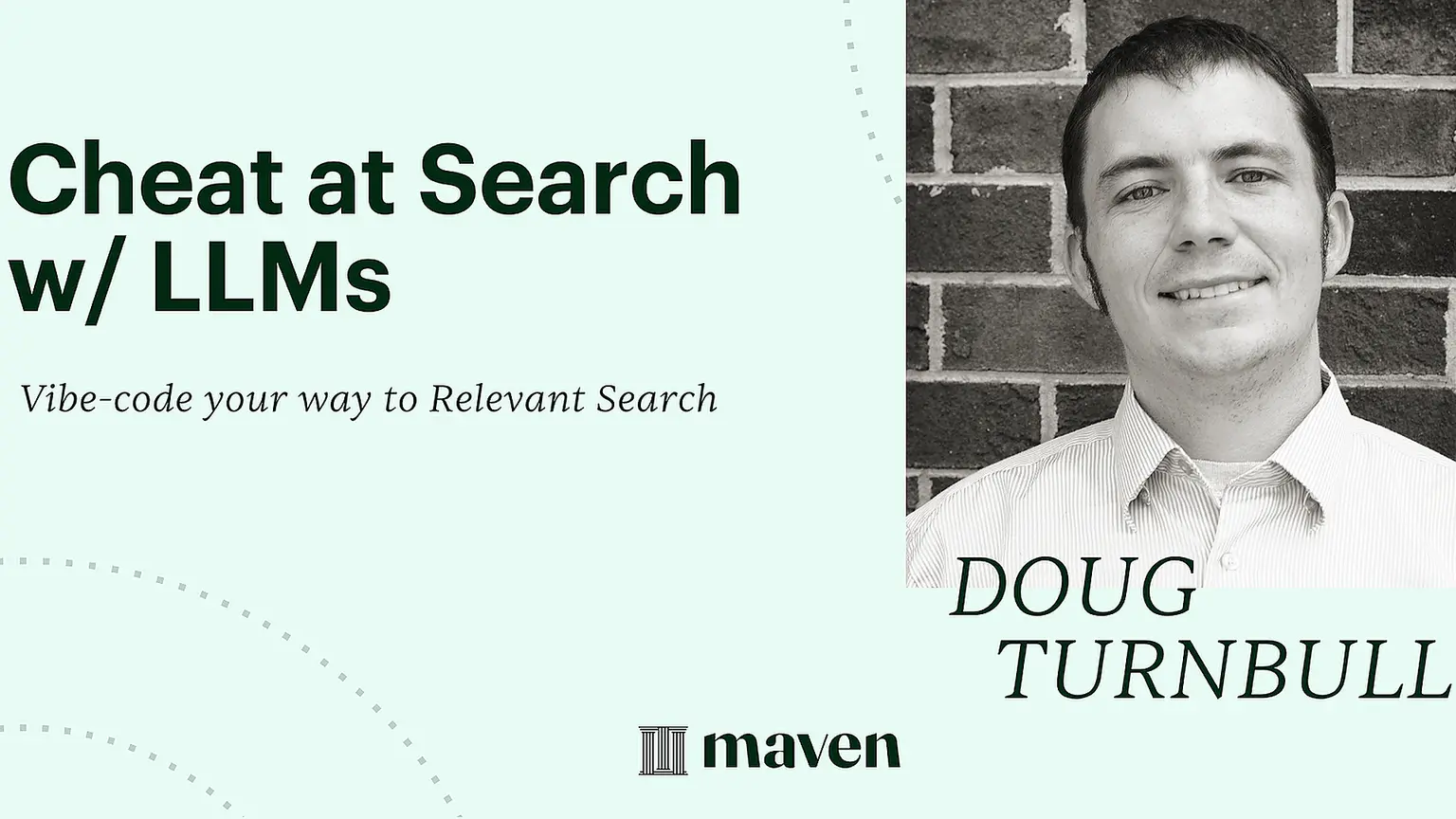